Parkinson's Disease Prediction
This project explores the use of machine learning to predict UPDRS motor symptoms in individuals with Parkinson's disease from force plates data.
Abstract
Parkinson’s disease (PD) is a neurodegenerative disease that affects motor abilities with increasing severity as the disease progresses. Traditional methods for diagnosing PD include a section where a trained specialist scores qualitative symptoms using the motor subscale of the Unified Parkinson’s Disease Rating Scale (UPDRS-III). The aim of this feasibility study was twofold. First, to evaluate quiet standing as an additional, out-of-clinic, objective feature to predict UPDRS-III subscores related to motor symptom severity; and second, to use quiet standing to detect the presence of motor symptoms. Force plate data were collected from 42 PD patients and 43 healthy controls during quiet standing (a task involving standing still with eyes open and closed) as a feasible task in clinics. Predicting each subscore of the UPDRS-III could aid in identifying progression of PD and provide specialists additional tools to make an informed diagnosis. Random Forest feature importance indicated that features correlated with range of center of pressure (i.e., the medial-lateral and anterior-posterior sway) were most useful in the prediction of the top PD prediction subscores of postural stability (r = 0.599; p = 0.014), hand tremor of the left hand (r = 0.650; p = 0.015), and tremor at rest of the left upper extremity (r = 0.703; p = 0.016). Quiet standing can detect body bradykinesia (AUC-ROC = 0.924) and postural stability (AUC-ROC = 0.967) with high predictability. Although there are limited data, these results should be used as a feasibility study that evaluates the predictability of individual UPDRS-III subscores using quiet standing data.
Project Images
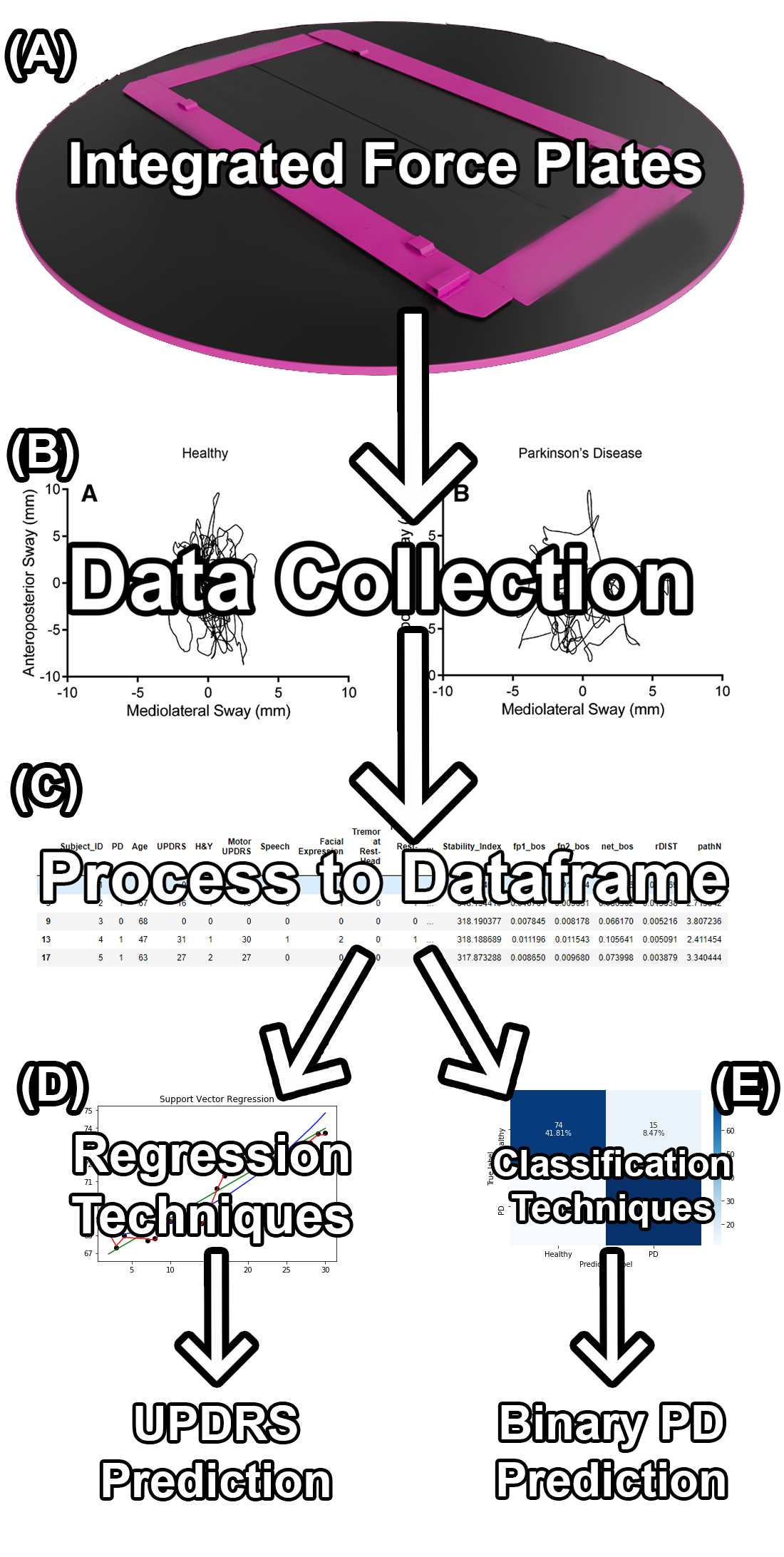
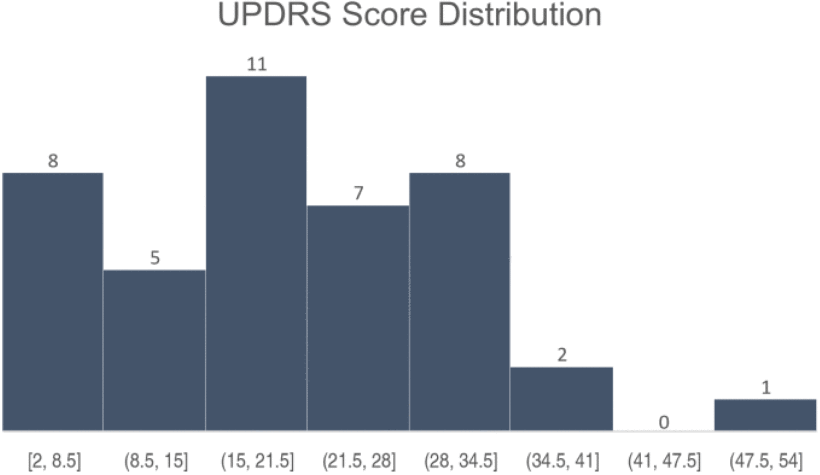
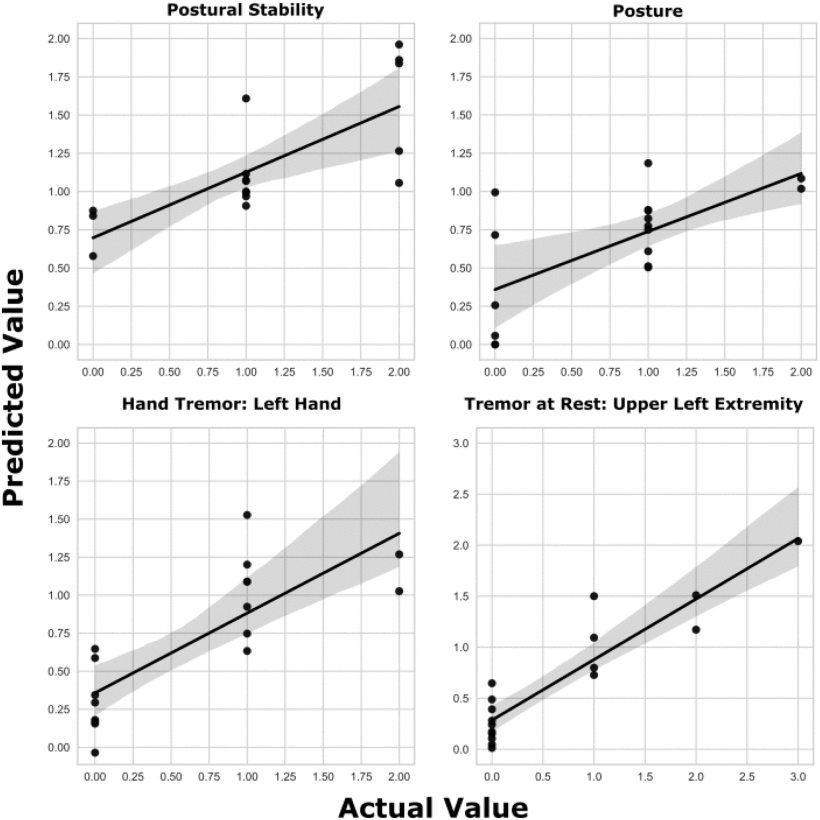
Conclusion
This study demonstrates the potential of using quiet standing force plate data combined with machine learning techniques to predict UPDRS-III motor symptoms in Parkinson’s disease patients. Further research with larger datasets and more diverse patient populations is necessary to validate and refine these predictive models.
References
2022
-
Predicting UPDRS Motor Symptoms in Individuals With Parkinson’s Disease From Force Plates Using Machine LearningIEEE Journal of Biomedical and Health Informatics, Jul 2022